

Tejas Ram
Allen High SchoolClass of 2023Allen, Texas
About
Projects
- "What Factors Statistically Separate the Best Tennis Players from the Rest Using Machine Learning" with mentor Tyler (July 15, 2022)
Project Portfolio
What Factors Statistically Separate the Best Tennis Players from the Rest Using Machine Learning
Started Mar. 10, 2022
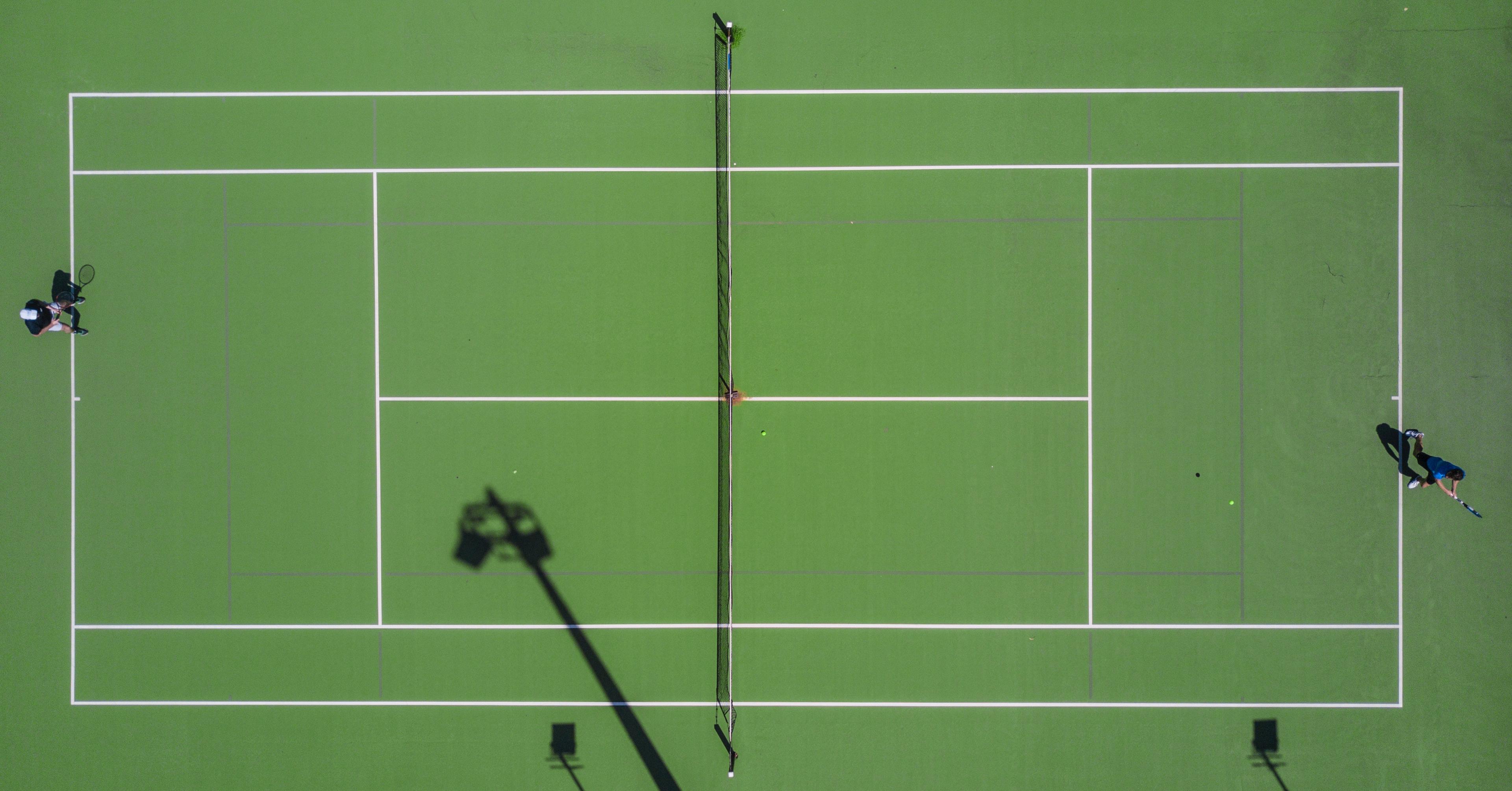
Abstract or project description
Throughout the years, tennis has seen the likes of many great athletes, such as Rod Laver, Boris Becker, and John McEnroe. Although these athletes were ahead of their time, changes in racket and string technology have changed the game altogether, making modern-day tennis almost completely different from the era of these great serve-and-volley players. At the start of the Open Era in 1968, rackets were predominantly wooden and strung with natural gut, but as new technologies emerged, these rackets grew larger and lighter, switching to a graphite frame with polyester strings. These changes allowed players to swing faster and hit bigger, introducing bigger serves, heavier forehands, and more physical baseline play.
Today, twenty-first century technology and innovations in both the tennis and medical fields have helped players develop incredible skill sets, with little difference in ability. In fact, despite a clear difference in match win percentage, data has shown that the top ranked ATP players win just slightly over 50% of their points. So what factors contribute to this rift in overall win percentage? To better understand the modern game, Python and its machine learning libraries can be used to identify differentiating factors between the best players in the world, and their less successful colleagues.
Question 1: What are the most important factors of a tennis match that separate the best players in the world from the rest of the ATP?
Recognizing such factors can help players at any level achieve improved success, by allowing them to target specific areas that are more crucial to winning tennis matches during their training sessions.
Question 2: Using match data and machine learning, can we make a model that accurately predicts a head to head winner between two ATP Players, based on statistics over the span of their careers?
After identifying the factors that distinguish more successful tennis players, we can use machine learning techniques to potentially create a model that predicts the winner of a head to head match, using data from the careers of both players.