Pose Estimation for Sprinter Form Comparisons
Project by Polygence alum Bryan
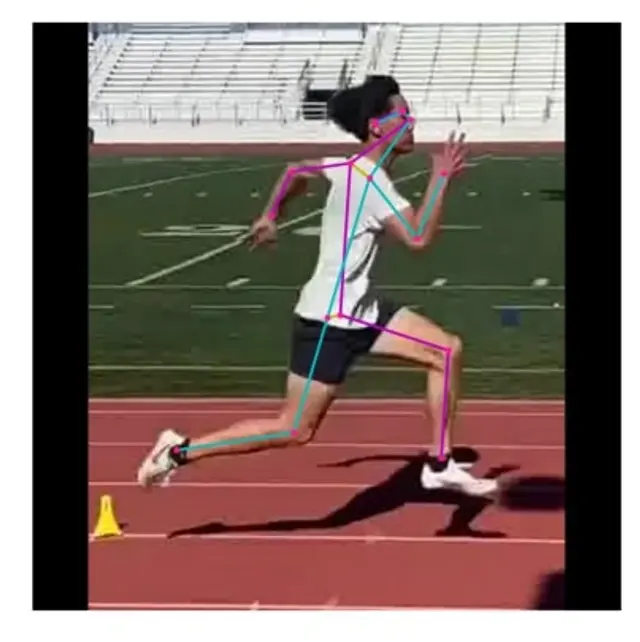
Project's result
We discovered that high schoolers tend to have a wider range of motion compared to professional athletes, who, at their top speed at maximal height projection, tend to show tighter body angles. Ground-to-air ratios have a somewhat positive correlation with sprinting speed: athletes who tend to have a lower ground-to-air ratio also have quicker split times. Our models showed that the front hip and elbows also tend to determine the speed of high school athletes.
They started it from zero. Are you ready to level up with us?
Summary
Our study aims to discover what joints and other factors play the most important role in developing faster sprinters. Using data from both high schoolers and professional athletes, we compared differences in each athlete's top speed position at their MVP stance, which is the maximal height of vertical projection as defined by the ALTOS Kinogram Method. With TensorFlow's MoveNet Thunder pose estimation model, we investigated each athlete's limb angles in the legs and arms. Alongside these angle key points, we measured the ground-to-air ratios (number of frames with foot on the ground divided by number of frames with foot in the air) of each athlete at their top speed. Comparing the angles, we discovered that professional athletes tend to have a more constricted range of movement at their maximal height projection while high school athletes tended to swing wider in the same position. With speed data of the high school athletes, we also discovered that a shorter ground-to-air ratio also meant a typically faster time. Paired with this data, we also found that the front hip and elbows tend to determine speed in athletes. However, while these findings may serve as benchmarks for success, performance will vary among athletes, so individualized training will most benefit a sprinter.

Atharv
Polygence mentor
MA Master of Arts
Subjects
Computer Science, Quantitative
Expertise
Data Science
Check out their profile

Bryan
Student
Graduation Year
2026
Project review
“I wanted to be able to merge my passion and a field I would like to study with Polygence, and I feel that the program has greatly helped me better understand how to work in my field.”
About my mentor
“Atharv is an amazing mentor; he will guide you through the whole process and set realistic goals while also being a good friend to talk to.”