Financial Market Sentiment Analysis Using Large Language Models (LLM) and Retrieval-Augmented Generation (RAG)
Project by Polygence alum Vivaan
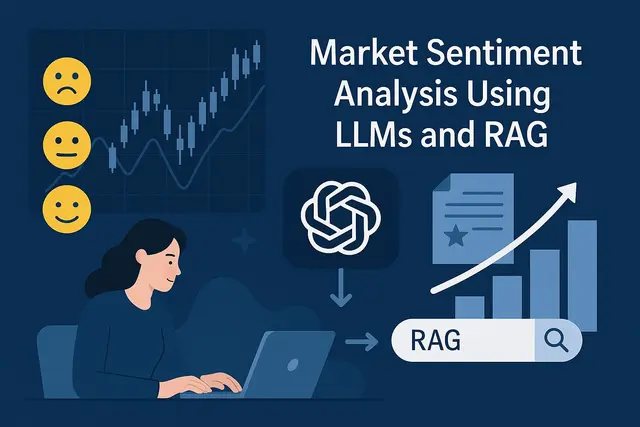
Project's result
This research demonstrates the significant potential of advanced machine learning models and retrieval augmented generation (RAG) systems for financial market sentiment analysis. By using FinBERT as the core language model, this study developed a framework to predict next-day stock price movements based on sentiment extracted from financial news articles. The data preprocessing and sentiment scoring methodologies show that sentiment scores have a measurable, albeit limited, impact on stock price fluctuations. The regression analysis indicates that sentiment explains approximately 1% of the variance in next-day stock price movements, suggesting that while sentiment is an important input, other factors such as market conditions, sector trends, and macroeconomic indicators play a significant role. The findings highlight that negative sentiment tends to have a stronger immediate impact on stock prices compared to positive sentiment. Despite challenges like data privacy concerns, algorithm biases, and the need for continuous model updates, sentiment analysis remains a valuable tool for investors and analysts. The integration of sentiment insights with traditional financial indicators offers a comprehensive approach to predicting stock movements and making informed investment decisions.
They started it from zero. Are you ready to level up with us?
Summary
The stock market is a complex, non-linear, and time-variant system heavily influenced by public sentiment. Understanding the sentiment behind market movements can offer invaluable insights for investors, hedge funds, and financial analysts. Market sentiment analysis involves using machine learning techniques to interpret and quantify the emotions and opinions expressed in textual data, such as news articles and financial reports.
Recent advancements in machine learning and natural language processing (NLP) have enabled the use of large language models (LLMs) to perform sentiment analysis more effectively. Specifically, fine-tuned models like FinBERT are well-suited for financial sentiment analysis due to their ability to interpret domain-specific language. These models, combined with a Retrieval-Augmented Generation (RAG) pipeline, provide a robust framework to retrieve relevant financial news articles, process them for sentiment classification, and correlate sentiment scores with historical stock prices.
The proposed methodology includes extensive data preprocessing, such as vector embedding generation using Sentence-BERT, to ensure the quality of the dataset. Sentiment scores from FinBERT are used to predict next-day stock price movements, with findings showing that negative sentiment has a stronger immediate effect on prices compared to positive sentiment. However, the regression analysis reveals that sentiment alone explains only about 1% of the variance in stock price movements, indicating the influence of other factors such as market conditions, sector-specific trends, and macroeconomic indicators.
This paper aims to assess whether sentiment can serve as a reliable indicator for short-term stock price movements and how it can enhance trading strategies. The research underscores the value of integrating sentiment analysis with other financial indicators, offering a comprehensive approach to understanding market dynamics. For the purposes of this study, short-term stock price change is defined as the price movement from the market close on the day of the news publication to the market close on the following trading day. Future considerations include expanding data sources, incorporating real-time data processing, and addressing challenges such as model bias and data privacy to improve the robustness of sentiment analysis systems.

Mohith
Polygence mentor
MS Master of Science
Subjects
Business, Quantitative, Computer Science, Engineering
Expertise
Business, Econ, App development, Web Development, AI/ML Algorithm development, other Computer Science areas, High School subjects
Check out their profile

Vivaan
Student
Graduation Year
2026
Project review
“The mentor was great and I really felt like I learned so much from this process!”
About my mentor
“Mohith is an amazing mentor who is extremely knowledgeable about AI/ML, LLM, Software Engineering and Systems design. His mentorship throughout this project has been invaluable, particularly in guiding me through the intricacies of building and fine-tuning language models and applying sentiment analysis techniques in a financial context. I am deeply grateful for his encouragement, expertise, and commitment to helping me refine my technical and analytical skills.”
Check out their profile